Group II - Final Project Report (1) (1)
.docx
keyboard_arrow_up
School
University Canada West *
*We aren’t endorsed by this school
Course
650
Subject
Business
Date
May 13, 2024
Type
docx
Pages
22
Uploaded by MateMoonYak12 on coursehero.com
1
Group II :- Final Project Report
Olaoluwa Opaola – 2206218
Kunal Mittal – 2231823
Parveen Kataria – 2226061
Pamela Hernandez – 2242246
Malvika Makwana – 2303922
Arjunkrishna – 2305298
Anureet Kaur Dhaliwal – 2243237
Bhawana Sharma Koirala - 2311669
University Canada West
BUSI 650-49 Business Analytics
Sana Ramzan
26
th March 2024
2
Introduction
The paper explains the regression analysis's outcomes on the financial data collected from both
Fraud and Non-Fraud Companies and the narration of the key financial indicators descriptive
analysis. Regression analysis is the tool to find out and describe the relationships inside financial
indicators and the descriptive analysis serves the purpose of understanding the distribution and
dispersion of these parameters. This analysis is performed by using predictive modelling
techniques that are used in fraud detection, namely: decision tree, multiple regression analysis,
clustering analysis, logistic regression, and neural networks. All the models are evaluated using
different metrics and one of them is the accuracy, precision, recall, support, and F1, (Yacouby,
R., & Axman, D., 2020). The main research question will be to figure out whether different
analytics techniques can be somehow used to detect fraudulent activities within the data set. In
order to answer these questions, I will conduct a number of sub-sections, for example: studying
the descriptive statistics of the dataset, using linear regression models, creating visualizations
using Tableau, and implementing predictive analytics using machine learning.
3
Brief Description of Techniques
Descriptive Statistics:
Descriptive analysis refers to the summarized information about the data,
which includes the measure of central tendency as well as the dispersion measure, and the
distribution, which is helpful to understand the characteristics of the data.
Linear Regression:
A basic linear regression model is applied to estimate the connection
between dependent and independent variables. In the light of this, the learning will help to know
how factors may contribute the occurrence of fraudulent practices.
Tableau Dashboard:
Tableau has the capability to create an interactive and informative
dashboard with the aim of delving deep into data visually and uncovering hidden trends or
patterns that would otherwise have remained untapped.
Predictive Analytics (Machine Learning):
Machine-learning algorithms like decision tree, logistic regression and neural networks can be used to identify fraudulent behavior on the basis of the patterns that are available from previous data.
Analytical Reports
Descriptive Report and Linear Regression:
This section will give a brief descriptive report of the dataset’s characteristics, and then it will
contain a linear regression analysis on the next five to study the connection between the
variables. The database will be completed on Excel, containing the main findings from
descriptive analysis and regression. The statistical analysis revealed various differences between
the groups and made outliers or extreme values in many ratios and performance indicators
4
visible, (Yacouby, R., & Axman, D., 2020). The tail events often exhibited leptokurtic behavior,
spanning equally to both sides, and were either positively or negatively skewed, based on the
direction of the tail events. However, the regression result was the highest among the dependent
variables with the return on assets as the independent variable (M-Score, Current Ratio, Quick
Ratio, Accounts Receivable Turnover, and Asset Turnover).
REGRESSION
The first multiple linear regression was created by selecting the data of Fraud companies, with
the dependent variable of Net Profit Margin, and the independent variables M score, Debt to
Equity, Quick Ratio, Accounts Receivable Turnover, and Return on Assets. The Multiple R
(0.13) shows a positive weak relationship and R Square (0.01769) a weak relationship between
Net Profit Margin and the independent variables. Meanwhile Adjusted R Square is negative (-
0.0216115) which is less than R Square, interpreted as invaluable.
Regression Statistics
Multiple R
0.1329709
R Square
0.01768126
Adjusted R Square
-0.0216115
Standard Error
987.252668
Observations
131
Regarding the relationship between the independent variable of Net Profit Margin with M Score,
Debt to Equity, Quick Ratio, Accounts Receivable turnover, and Return on Assets, since the P-
5
value is greater than the significance level of 0.05, the relationship with the net profit margin of
the fraud companies confirms as statistically insignificant.
P-value
Intercept
0.9825640
9
MScore
0.8732479
7
Debt to Equity
0.9951125
9
Quick Ratio
0.1448416
Accounts
Receivable
Turnover
0.9989029
6
Return on Assets
0.8675988
5
The second multiple linear regression was generated by selecting the same dependent variable
but only of the Non-Fraud companies, and the independent variables, Mscore, Debt to Equity,
Quick Ratio, Accounts Receivable Turnover, and Return on Assets. The Multiple R
(0.17) shows
a positive weak relationship and R Square (0.031) a weak relationship between Net Profit
Margin and the independent variables. Also, Adjusted R Square (-0.027) is invaluable as it is less
value than R Square.
Regression Statistics
Multiple R
0.176581246
6
R Square
0.031180936
Adjusted R Square
0.027816981
Standard Error
92.05339107
Observations
1446
The results of the P-value of these variables is greater than 0.05, meaning again the relationship
is statistically insignificant between the data of the Non-Fraud Companies.
P-value
Intercept
0.257399283
MScore
1.96937E-07
Debt to Equity
0.597260405
Quick Ratio
0.053579691
Accounts
Receivable
Turnover
3.11573E-05
Return on Assets
0.51247816
The third multiple linear regression was with the selection of Mscore data only of Fraud
companies as the dependent variable and the independent Variables of Gross Profit Margin, Debt
on Equity, Current Ratio, EBITDA, and Return on Equity. The Multiple R
(0.024) shows a
positive weak relationship and R Square
(0.00059) a weak relationship between Net Profit
Margin and the independent variables. Also, Adjusted R Square
(-0.0393) is invaluable as it is
less value than R Square.
Regression
Your preview ends here
Eager to read complete document? Join bartleby learn and gain access to the full version
- Access to all documents
- Unlimited textbook solutions
- 24/7 expert homework help
Related Questions
Q1: Define Project Management plan with respect to the following points?
· The role of the implementation agency
· Beneficiary participation
· Organizational structure and staffing
· Financial management
· Time control and remedial action
· Supervision of the implementation of the project schedule
· Supervision of implementation of a project management
arrow_forward
The expected times and variances for the
activities for a project for Test Marketers, Inc.
are given below. (Assume time is provided in
days.)
ID
12345678
Description
Pilot production
Select channels of distrib.
Develop mktg. program
Test market
Patent
Full production
Ad promotion
Release
Predecessor
None
None
None
1
1
4
3
2,5,6,7
t₂
6
7
4
4
10
16
3
2
Varlance
[(b-a)/6]²
34225221
10
arrow_forward
quetion 1
a)What is a burn-down chart? What purpose does it serve?
b)Why should a project manager be concerned with monitoring a project’s progress?
c) Describe how the SPI and CPI can be used to forecast the final cost of a project?
arrow_forward
Project comunication plan for NASA s moon landing project
arrow_forward
You are assigned as a project manager to lead a new quality improvement project.
Q Management is asking for a project management plan. Which of the following should
you aeate FIRSTI
A
A process improvement plan
A quality management plan
CA project scope statement
D A project management plan
41
193
retu
AB
arrow_forward
Project Implementation Plan for Culinary Tourism.:
• You will be creating a Project Implementation Plan for the new service to be offered by your team/group
• Step 4 - Outline of Deliverables - Deliverables are the tangibles of your company. Outlining the deliverables, you hope to create can serve as a resource. This will include time frames/ due dates for ensuring the product/ service is brought to the market. For example - you can use the GANTT CHART for this part (GANTT chart helps teams to plan work around deadlines and properly allocate resources). You are also required to assign tasks for team members for which they would be accountable, and allocate resources for each input required.
arrow_forward
Ć D 4. In the title: EFFECT OF PARTICIPATION IN INTERSCHOOL CONTEST ON SENIOR HIGH SCHOOL
STUDENT'S ACADEMIC PERFORMANCE, the population involved in the study is
A. GAS students of SHS
B. All participating class
C. TVL class
D. SHS Participating Students
ainiog E eiT to sons
auneino
niob m'ltery
ABCD 5. In the title: STCNHS SCHOOL-WIDE LANDSCAPING PROJECT: ITS EFFECT ON
ATTITUDES AND PERCEPTION OF PARENTS
The scope of the study is
A. STCNHS
B. Schools Division of Occidental Mindoro
C. STCNHS - Senior High School
D. STCNHS - Junior High School
90plent cuta0199
arrow_forward
Mini Case Study - EAP
Mary Jones was recently appointed to the new position of director of employee assistance programs at Mega Hotels. Over the objections of her boss, the director of human resources, Mary quickly instituted an alcohol and drug abuse awareness program in each of the hotel’s properties. Her boss opposed the program on the basis that Mega Hotels knew little about the kinds of problems its employees faced.
Mary established in-house programs at each property in the chain and hired specialists to implement and supervise the programs. The annual salaries of the five specialists averaged $24,000.
Three months later, the president of Mega Hotels asked for a status report on the employee assistance program. At that time, Mary could report only a five percent employee participation rate. The president was disappointed with this rate and threatened to cancel the program. The president asked Mary to evaluate the progress of the program and to make recommendations for its…
arrow_forward
RESEARCH TOPICS1. The difference between project feasibility and project appraisal and their significance toproject sustainability2. The interplay between the project components (Cost, time, scope and quality) and thesignificance to project performance and sustainability3. Report writing process and utility of project report to project management
arrow_forward
(Use the information below to solve Q11 - Q20)
Given the following information regarding a project:
Predecess
ors
None
None
a
a
b
b
Activity
a
b
C
d
e
CD
f
OC
g
h
Duration
3
1
3
4
4
5
23
c, e
f
Which of the following is NOT a path in the network?
O a-d
O a-c-g
b-c-g
O b-e-g
b-f-h
arrow_forward
From Chapter 3 - Read, analyze, compare/contrast case study 1: JWD consulting's project management intranet site project (predictive/waterfall approach) and case study 2: JWD consulting's project management intranet site project (agile approach).
arrow_forward
23 The Identify Stakeholders process has four inputs Three of them are project
charter, enterprise environmental factors and organizational process assets. What
is the fourth one?
A Procurement documents
B Stakeholder register
C. Stakeholder engagement matrix
D Stakeholder management plan
24 The Project Managers have maximum authority in which type of organization?
A Weak Matrix
B Strong Matrix
C Functional
D Balanced Matrix
25 Accepted deliverables are an input to the Close Project or Phase process
These deliverables would have been accepted through which of the following
processes?
A. Validate Scope
B Control Quality
C. Control Scope
D. Perform Quality Assurance
26. Which process group corresponds to the "do" part of the plan-do-check-act
cycle?
A Closing
B. Monitoring and Controlling
C. Planning
D. Executing
27. To control the schedule, a project manager is re-analyzing the project to
predict project duration. This is done by analyzing the sequence of activities with
the least…
arrow_forward
1. A Company achieved client success by improving its processes and increasing its consistency in project delivery. Explain achieving project success of projects in an organisation.
arrow_forward
https://www.space.com/nasa-picksspacex-blue-origin-private-moon-lander-companies.html
Project Managers are often tasked with the responsibility to select potential projects and to motivate their selection to senior management in order to procure commitment of funds and resources.1 Establish and highlight the importance of project management as a key enabler of organisational strategy and identify how this relates to NASA as per the case study.2 Discuss the project selection criteria available to Project Managers and elaborate on NASA’s approach to project selection for future moon missions.
arrow_forward
Project Implementation Plan for Culinary Tourism.
• You will be creating a Project Implementation Plan for the new service to be offered by your team/group
• Step 4 - Outline of Deliverables - Deliverables are the tangibles of your company. Outlining the deliverables, you hope to create can serve as a resource. This will include time frames/ due dates for ensuring the product/ service is brought to the market. For example - you can use the GANTT CHART for this part (GANTT chart helps teams to plan work around deadlines and properly allocate resources). You are also required to assign tasks for team members for which they would be accountable, and allocate resources for each input required.
Kindly prepare a Conclusion for Culinary Tourism
State main points and way forward in the future.
Synthesize the entire paper with specific details as included in the paper
No new information is included in the conclusion
arrow_forward
AABCDE
Activity
J
К
Predecessor
None
PZABUD
FGHL-KL
EOFICI
LA
C
H
с
H
J.I,K
Draft PDS
Identify team
Kickoff team meeting
Source previous rocket
Identify & order missing
or new materials/parts
Assembly
Simulation
Alpha Test
Beta Test
Training of key people
Buy food to bring
Launch
DITONT
Duration (wks)
1
1
0
2
4
8722860
1. Show the Critical Path. Give reasons for your answer.
2. If you needed to shorten the duration of the project, which activity or activities would you shorten and why?
arrow_forward
What is the minimum cost of crashing the following project at Sawaya Robotics by 4 days?
NORMAL
CRASH
TIME (DAYS) TIME (DAYS)
9
7
7
6
5
4
6
4
9
6
ACTIVITY
Design (A)
Wiring (B)
Chip install (C)
Software (D)
Testing (E)
The minimum cost of crashing the following project at Sawaya Robotics by. 4 days is $
NORMAL
COST
200
800
600
1,100
1,200
CRASH
COST
300
875
675
1,300
1,875
IMMEDIATE PREDECESSOR(S)
(enter your response as a whole number).
A, B
C
arrow_forward
Give examples of the following strategies (project where they can be used) • Scenarios
arrow_forward
Given the data table below develop the precedence network and perform the CPM calculations. Then, using the proposed crashing strategy calculate
the normal, least-cost, and crash duration for the project below. Calculate the cost associated with each duration. Indirect cost (overhead) is $120 per
day.
Activity IPA
A
B
E
F
G
H
|
J
A
A,B
B
C,D
F
E,G
H,I
Duration
Cost $
Normal Crash Normal Crash
5
4
2
4
7
4
6
8
A
3
3
4
5
3
4
3
2
$770 $900
$660
$700
$800
$1,070
$1,000
$1,110
$800
$920
$560 $630
$700
$810
$1,000 $1,260
$500
$580
$400 $600
arrow_forward
CASE – CAPACITY BUILDING PROJECT AIMS TO INCREASE YOUTHINVOLVEMENT IN SUSTAINABLE DEVELOPMENTBasseterre, St. Kitts, April 29, 2021 (SKNIS): St. Kitts and Nevis Youth Ambassador Corpsofficially launched a new project on Wednesday (April 28, 2021) designed to increase thecontributions of young people to the sustainable development of the twin-island Federation.The initiative, dubbed the Youth Capacity Building Small Grants Project on Youth in SustainableDevelopment, will build the capacity and practical skills of young people to design, implement,and monitor interventions relevant to the social, economic, and sustainable development of youthand their communities. The project is geared towards members of youth groups specifically. It isspearheaded by the national youth ambassador corps in collaboration with the GEF Small GrantsProgramme (SGP), and the Departments of Youth in St. Kitts, and Nevis.The Small Grants Project is funding the initiative to the tune of US$49,970.00. SGP…
arrow_forward
CASE – CAPACITY BUILDING PROJECT AIMS TO INCREASE YOUTHINVOLVEMENT IN SUSTAINABLE DEVELOPMENTBasseterre, St. Kitts, April 29, 2021 (SKNIS): St. Kitts and Nevis Youth Ambassador Corpsofficially launched a new project on Wednesday (April 28, 2021) designed to increase thecontributions of young people to the sustainable development of the twin-island Federation.
The initiative, dubbed the Youth Capacity Building Small Grants Project on Youth in SustainableDevelopment, will build the capacity and practical skills of young people to design, implement,and monitor interventions relevant to the social, economic, and sustainable development of youthand their communities. The project is geared towards members of youth groups specifically. It isspearheaded by the national youth ambassador corps in collaboration with the GEF Small GrantsProgramme (SGP), and the Departments of Youth in St. Kitts, and Nevis.
The Small Grants Project is funding the initiative to the tune of US$49,970.00. SGP…
arrow_forward
Case: Friendly Assisted Living Facility-3 (pg.104)
Q1: Define the deliverables for this project
Q2: Define the constraints and assumptions for this project
Q3: Develop a preliminary Level 1 WBS
Q4: Develop a RACI matrix for this project based on the level 1 WBS
Q5: Would a Project Charter have been useful here? Explain.
C
A
S
E
Friendly Assisted Living Facility-3
Continuing with the ALF Steering Committee meeting Fred led a discussion of all the major steps that must be included in the project plan, and inked each team member to identify the areas for which they would accept responsi bility. The hospital's Construction Project Manager took responsibility for the constructions of the facility, and the COO volunteered to oversee the building design, as well as define the needs for food services, housekeeping, staff ing, and policy and procedure development. The CFO agreed to develoys the budgets for each area of the project
as well as the operating budget for the facility.…
arrow_forward
How Can You Get to a Backlog of over 100 Projects?
There are never enough resources to get everything done." Backlogs build over time. Sacred cow
projects get included in the selection system. Projects proposed from people who have left the airline stil
reside in the project portfolio. Non-value-added projects somehow make their way into the project
portfolio. Soon the queue gets longer. With everyone in IT working on too many projects concurrently,
project completion and productivity are slow.
Which Projects Remain?
To cut the number of projects, the steering committee used a weighting scheme that reflected the airline's
priorities, which were: fly safe, generate revenue, reduce costs, and customer service. The weighting
scheme easily weeded out the fluff. Coady noted that "by the time you get to the 20s the margin of
differentiation gets narrower and narrower." Of the remaining projects, project sponsors had to have solid
justification why their project is important. Reduction of the…
arrow_forward
Q4) What Is Project Quality Management? Write briefly about the five Cost Categories Related to Quality .Describe about Quality Assurance.
arrow_forward
3.8 Roger Ginde is developing a program in supply chain management certification for managers. Ginde has listed a num- ber of activities that must be completed before a training program of this nature could be conducted. The activities, immediate pre- decessors, and times appear in the accompanying table:
a) DrawtheappropriateAONPERTdiagramforJ.C.Howard’s management team.
b) Find the critical path.
c) What is the project completion time?
• • 3.11 The following is a table of activities associated with a project at Rafay Ishfaq’s software firm in Chicago, their dura- tions, and what activities each must precede:
ACTIVITY
DURATION (WEEKS)
PRECEDES
A (start)
1
B, C
B
1
E
C
4
F
E
2
F
F (end)
2
—
ACTIVITY
IMMEDIATE PREDECESSOR(S)
TIME (DAYS)
A
—
2
B
—
5
C
—
1
D
B…
arrow_forward
Project Management
FAO-Funded Aquaponics Project Launched In Barbados
Barbados GIS – The United Nations Food and Agriculture Organization (FAO) has collaborated with the Ministry of Maritime Affairs and the Blue Economy, and local business. Adams Aquafarms, on a project to train persons in aquaponics.
Aquaponics is a sustainable way of integrating land-based fish farming with plant production. Both are placed in a tank, and the set up allows for them to coexist, with the waste produced by the fish fertilizing the plants, while the plants purify the water for the fish.
The training, funded by the FAO, will take place at a demonstration facility, located at Adams Aquafarms, Hopewell, St. Thomas. The FAO is currently targeting 30 entrepreneurs and 10 teachers.
Minister of Maritime Affairs and the Blue Economy, Kirk Humphrey, believes the project will be “transformative”, as it has the potential to empower Barbadians.
“In aquaponics, you have the opportunity to have, at the same time,…
arrow_forward
Project definition involves: Your answer:
1-identifying geo-social environment, econo-political environment, business environment, and internal environment.
2- identifying stakeholders, understanding their motivations,and prioritising and managing different stakeholders.
3- determining objectives, scope. and strategy.
4- Defining operations
arrow_forward
How can a project incorporate sustainability
arrow_forward
States)
B
E-
Text Predictions: On
Activity
A
I U
2. Given below are additional details for the Evergreen Company's construction project, i.e.
three time estimates.
B
C
D
ALL
E
F
Optimistic
0.5
ARDCCDGEe
Normal
2.5
1
0.9
0.5
Most Likely
1
2
3
2
1
AaBb CcDdEe
No Spacing
1
AaBbCcL AaBbCcDd.
Heading 1
Heading 2
Pessimistic
2
2.5
3.5
3
1.1
1.5
a) Calculate the expected completion times and completion variances for each activity.
b) What are the expected days to completion now for Evergreen?
c) Compute the variance and standard deviation of the Evergreen project's completion time.
d) What is the probability that the Evergreen Company's project can be completed in 6.5
days?
e) What would be Evergreen Company's completion days if we needed a 95% confidence
level?
f) Which noncritical activities should Evergreen Company monitor closely? Why?
Focus E
arrow_forward
15. For the network shown:
Crash Cost/week - (CC-NC)/(NT-CT)
TOTAL COST-$44,000
Crash D-$1,000
Crash G-$2,000
Crash A-$3,000
0
0
ATS)
Activity
A
B
54
C
D
E
F
45
45
B(10)
C(8)
1514
15
10
13 12
14
Normal Time
5
8
6
7
14 15
15
D(6)
21 20 19
21
a. Determine the critical path and the early completion time in weeks for the project.
b. For the data shown, reduce the project completion time by three weeks. Assume a lin-
ear cost per week shortened, and show, step by step, how you arrived at your schedule.
20 19
E(7)
14
13 17
F(4)
17 21
Normal Cost
$7,000
12,000
5,000
4,000
3,000
6,000
7,000
21
20
21 25
G(4)
25
Crash Time
3
7
7
24 23 22
A 2
B3
C1
D1
E1
5
6 F1
3 G1
Crash Cost
$13,000
18,000
7,000
5,000
6,000
7,000
9,000
A $3,000
B $2,000
C $2,000
D $1,000
E $3,000
F $1,000
G $2,000
arrow_forward
Project Management in Practice 4EDISCUSSION QUESTIONDQContrast the three types of nonnumeric project selection methods. Could any specific case combine two of them, such as the sacred cow and the oper- ating necessity, or the comparative benefits and the competitive necessity?
arrow_forward
SEE MORE QUESTIONS
Recommended textbooks for you
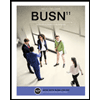
BUSN 11 Introduction to Business Student Edition
Business
ISBN:9781337407137
Author:Kelly
Publisher:Cengage Learning
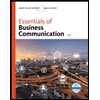
Essentials of Business Communication (MindTap Cou...
Business
ISBN:9781337386494
Author:Mary Ellen Guffey, Dana Loewy
Publisher:Cengage Learning
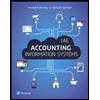
Accounting Information Systems (14th Edition)
Business
ISBN:9780134474021
Author:Marshall B. Romney, Paul J. Steinbart
Publisher:PEARSON
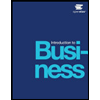
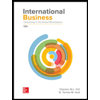
International Business: Competing in the Global M...
Business
ISBN:9781259929441
Author:Charles W. L. Hill Dr, G. Tomas M. Hult
Publisher:McGraw-Hill Education
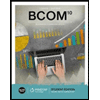
Related Questions
- Q1: Define Project Management plan with respect to the following points? · The role of the implementation agency · Beneficiary participation · Organizational structure and staffing · Financial management · Time control and remedial action · Supervision of the implementation of the project schedule · Supervision of implementation of a project managementarrow_forwardThe expected times and variances for the activities for a project for Test Marketers, Inc. are given below. (Assume time is provided in days.) ID 12345678 Description Pilot production Select channels of distrib. Develop mktg. program Test market Patent Full production Ad promotion Release Predecessor None None None 1 1 4 3 2,5,6,7 t₂ 6 7 4 4 10 16 3 2 Varlance [(b-a)/6]² 34225221 10arrow_forwardquetion 1 a)What is a burn-down chart? What purpose does it serve? b)Why should a project manager be concerned with monitoring a project’s progress? c) Describe how the SPI and CPI can be used to forecast the final cost of a project?arrow_forward
- Project comunication plan for NASA s moon landing projectarrow_forwardYou are assigned as a project manager to lead a new quality improvement project. Q Management is asking for a project management plan. Which of the following should you aeate FIRSTI A A process improvement plan A quality management plan CA project scope statement D A project management plan 41 193 retu ABarrow_forwardProject Implementation Plan for Culinary Tourism.: • You will be creating a Project Implementation Plan for the new service to be offered by your team/group • Step 4 - Outline of Deliverables - Deliverables are the tangibles of your company. Outlining the deliverables, you hope to create can serve as a resource. This will include time frames/ due dates for ensuring the product/ service is brought to the market. For example - you can use the GANTT CHART for this part (GANTT chart helps teams to plan work around deadlines and properly allocate resources). You are also required to assign tasks for team members for which they would be accountable, and allocate resources for each input required.arrow_forward
- Ć D 4. In the title: EFFECT OF PARTICIPATION IN INTERSCHOOL CONTEST ON SENIOR HIGH SCHOOL STUDENT'S ACADEMIC PERFORMANCE, the population involved in the study is A. GAS students of SHS B. All participating class C. TVL class D. SHS Participating Students ainiog E eiT to sons auneino niob m'ltery ABCD 5. In the title: STCNHS SCHOOL-WIDE LANDSCAPING PROJECT: ITS EFFECT ON ATTITUDES AND PERCEPTION OF PARENTS The scope of the study is A. STCNHS B. Schools Division of Occidental Mindoro C. STCNHS - Senior High School D. STCNHS - Junior High School 90plent cuta0199arrow_forwardMini Case Study - EAP Mary Jones was recently appointed to the new position of director of employee assistance programs at Mega Hotels. Over the objections of her boss, the director of human resources, Mary quickly instituted an alcohol and drug abuse awareness program in each of the hotel’s properties. Her boss opposed the program on the basis that Mega Hotels knew little about the kinds of problems its employees faced. Mary established in-house programs at each property in the chain and hired specialists to implement and supervise the programs. The annual salaries of the five specialists averaged $24,000. Three months later, the president of Mega Hotels asked for a status report on the employee assistance program. At that time, Mary could report only a five percent employee participation rate. The president was disappointed with this rate and threatened to cancel the program. The president asked Mary to evaluate the progress of the program and to make recommendations for its…arrow_forwardRESEARCH TOPICS1. The difference between project feasibility and project appraisal and their significance toproject sustainability2. The interplay between the project components (Cost, time, scope and quality) and thesignificance to project performance and sustainability3. Report writing process and utility of project report to project managementarrow_forward
- (Use the information below to solve Q11 - Q20) Given the following information regarding a project: Predecess ors None None a a b b Activity a b C d e CD f OC g h Duration 3 1 3 4 4 5 23 c, e f Which of the following is NOT a path in the network? O a-d O a-c-g b-c-g O b-e-g b-f-harrow_forwardFrom Chapter 3 - Read, analyze, compare/contrast case study 1: JWD consulting's project management intranet site project (predictive/waterfall approach) and case study 2: JWD consulting's project management intranet site project (agile approach).arrow_forward23 The Identify Stakeholders process has four inputs Three of them are project charter, enterprise environmental factors and organizational process assets. What is the fourth one? A Procurement documents B Stakeholder register C. Stakeholder engagement matrix D Stakeholder management plan 24 The Project Managers have maximum authority in which type of organization? A Weak Matrix B Strong Matrix C Functional D Balanced Matrix 25 Accepted deliverables are an input to the Close Project or Phase process These deliverables would have been accepted through which of the following processes? A. Validate Scope B Control Quality C. Control Scope D. Perform Quality Assurance 26. Which process group corresponds to the "do" part of the plan-do-check-act cycle? A Closing B. Monitoring and Controlling C. Planning D. Executing 27. To control the schedule, a project manager is re-analyzing the project to predict project duration. This is done by analyzing the sequence of activities with the least…arrow_forward
arrow_back_ios
SEE MORE QUESTIONS
arrow_forward_ios
Recommended textbooks for you
- BUSN 11 Introduction to Business Student EditionBusinessISBN:9781337407137Author:KellyPublisher:Cengage LearningEssentials of Business Communication (MindTap Cou...BusinessISBN:9781337386494Author:Mary Ellen Guffey, Dana LoewyPublisher:Cengage LearningAccounting Information Systems (14th Edition)BusinessISBN:9780134474021Author:Marshall B. Romney, Paul J. SteinbartPublisher:PEARSON
- International Business: Competing in the Global M...BusinessISBN:9781259929441Author:Charles W. L. Hill Dr, G. Tomas M. HultPublisher:McGraw-Hill Education
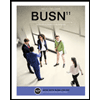
BUSN 11 Introduction to Business Student Edition
Business
ISBN:9781337407137
Author:Kelly
Publisher:Cengage Learning
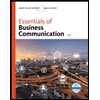
Essentials of Business Communication (MindTap Cou...
Business
ISBN:9781337386494
Author:Mary Ellen Guffey, Dana Loewy
Publisher:Cengage Learning
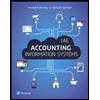
Accounting Information Systems (14th Edition)
Business
ISBN:9780134474021
Author:Marshall B. Romney, Paul J. Steinbart
Publisher:PEARSON
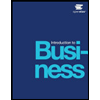
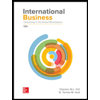
International Business: Competing in the Global M...
Business
ISBN:9781259929441
Author:Charles W. L. Hill Dr, G. Tomas M. Hult
Publisher:McGraw-Hill Education
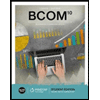